Environmental intelligence refers to the ability to gather, analyze, and refine information about the environment to help stakeholders make informed decisions and take effective action. It involves understanding the complexities of ecological systems, as well as the social, economic, and political factors that impact them.
“In the simplest of terms, environmental intelligence is the use of machine learning algorithms and other artificial intelligence (AI) algorithms to solve problems of prediction modeling and control of environmental systems, where systems refer to a broad number of fields of science such as hydrology food and energy, and climate science,” says Guido Ascenso, of the Politecnico of Milan, when talking at the CMCC’s webinar on Climate Intelligence.
In the real world, this can be seen in things such as advanced sensors and monitoring systems which are continuously providing real-time data on environmental conditions, such as air quality or water quality. Processing these vast amounts of data effectively can, in turn, allow decision-makers to identify emerging environmental risks and take proactive measures to address them.
AI and machine learning
Environmental intelligence covers a combination of different technologies that work together in three main phases. Firstly, it refers to technologies that help source data from the environment, both in situ and ex situ. This can be things like sensors or satellite data, for example. The second phase is to work on this data and elaborate on and process it. It is what is commonly known as data science. Finally, the data has to be then used to tackle a given environmental problem or help develop a policy that is data-driven, explains Francesco Matteucci, of the European Innovation Council during the CMCC webinar on climate intelligence webinar on climate intelligence.
“These are three stepping stones to environmental intelligence, and the overarching structure is the environment and using data to improve air, water and soil,” explains Matteucci.
Machine learning algorithms and big data analytics are central to this process as they can be used to speed up the process behind identifying patterns and trends in environmental data and hence enable decision-makers to develop more effective strategies to manage natural resources, reduce waste, and mitigate environmental impacts, for example.
“Artificial intelligence helps us to amplify and speed up the scientific process by working with a larger amount of data and automatically extracting possible connections or links between variables and processes,” says Andrea Castelletti, professor of Natural Resources Management at Politecnico di Milano, Italy, as well as lab head at the Environmental Intelligence Lab and coordinator and lead of Climate Intelligence (CLINT).
AI in climatology
“We need machine learning algorithms because, to this day, our understanding of climate processes is incomplete and physical-based models at times fail at predicting extreme events both in time and space, particularly when it comes to rare and extreme events,” says Ascenso. “Observational data sets have gaps which can make analysis hard. So, instead of accepting that this is the data we have to work with, we developed a machine learning model that fills in the gaps,” says Ascenso when talking about the CLINT project.
“Machine learning can then replace some of these missing components and integrate these models so that they can solve tasks where there is, in fact, enough data available.”
More specifically, AI and machine learning are being used in climate modeling, which involves simulating the interactions between various components of the Earth system, such as the atmosphere, oceans, and land surface. These models are essential for understanding the impact of climate change on different regions and developing effective mitigation and adaptation strategies both at a local and global scale.
Giovanni Coppini, CMCC scientist and head of the Ocean Prediction and Application research, looks at this connection of local and global issues with reference to oil spill forecasting and environmental indicators. The WITOIL (Where Is The Oil) service provides predictions of transport and transformation of actual or hypothetical oil spills in the Mediterranean Sea.
“A couple of years ago, there was a big accident in a refinery, and the oil spill started to pollute the Syrian Coast,” explains Coppini. “It’s hard to predict where the oil will go, but with satellite detection and then our automatic modelling system, using the highest resolution model available, we created a forecast of the pattern of the oil for the next 48 hours.”
Another example comes from the CLINT project: researchers are looking at developing an AI framework to process big climate datasets for improving climate science in the detection, causation, and attribution of extreme events, namely tropical cyclones, heatwaves and warm nights, droughts, and floods. The AI framework will cover the quantification of impacts on a variety of socio-economic sectors and across different spatial scales (from European to local hotspots in Africa and Europe), under historical, forecasted, and projected climate conditions.
The project then takes the process one step further by developing innovative AI-enhanced climate services into Web Processing Services and a demonstrator that will facilitate the uptake of project results by public and private entities for research and sector-specific services.
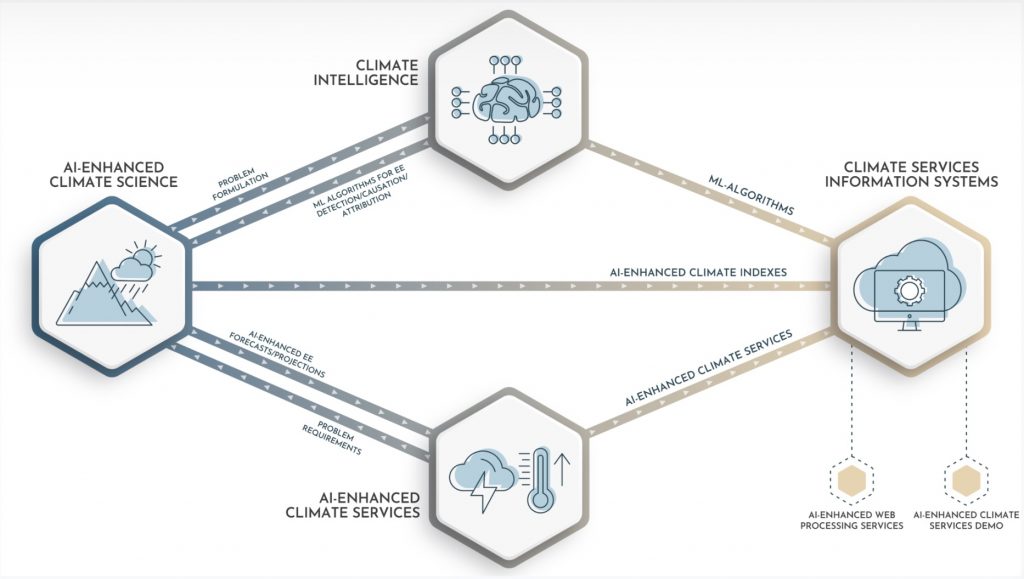
Environmental intelligence is therefore useful also in climate impact assessments, which involves evaluating the risks and vulnerabilities of different sectors and regions to climate change. For example, AI and machine learning can be used to analyze the impact of extreme weather events on infrastructure, agriculture, and public health and to identify the most effective adaptation strategies.
Converting quantity into quality
As artificial intelligence (AI) and machine learning are increasingly applied in climate change studies to analyze complex data sets, simulate and predict climate patterns, and help inform policy decisions, there is also a need to make sure that the outputs they generate lead to operational value for decision-makers.
“When you are involved in making decisions on critical issues, such as managing a given climate emergency, operational value of data allows you to give more accurate information to decision-makers on the ground,” says Castelletti.
“Today, in the climate and environmental field, we have an availability of data that was unthinkable even just twenty years ago. This has presented an opportunity as it lends itself to using AI to explore climate issues and find solutions. We now need to understand how to exploit this information and how to develop fast and effective control and optimization policies so that we can use it effectively.”
At the EOLIANN project, co-founder and chief product officer Emidio Granito is working to develop climate resilience modeling that helps institutions and businesses predict the likelihood and impact of climate risks, leveraging satellite data and using machine learning algorithms.
“As natural disasters increase, they are also becoming harder to predict, and there is huge variability which leads to large economic losses,” says Granito, who also explains that this can have severe implications on a variety of actors. “For banks and insurance companies not being able to estimate the impact of these extreme events correctly leads to a lot of financial losses due to underestimation of impacts.”
These companies typically rely on historical data, but Granito believes using AI and satellite data instead can provide better predictions of extreme events. “We are in a special time where a lot of data is being produced from earth observations from over 1,000 satellites and over 150 terabytes of data produced daily,” says Granito. “We want to use this data to provide accurate insight for decision makers.”
Environmental Intelligence – watch the CMCC webinar
Cover image of the article by rawpixel.com